Methods and techniques used in skill development through education
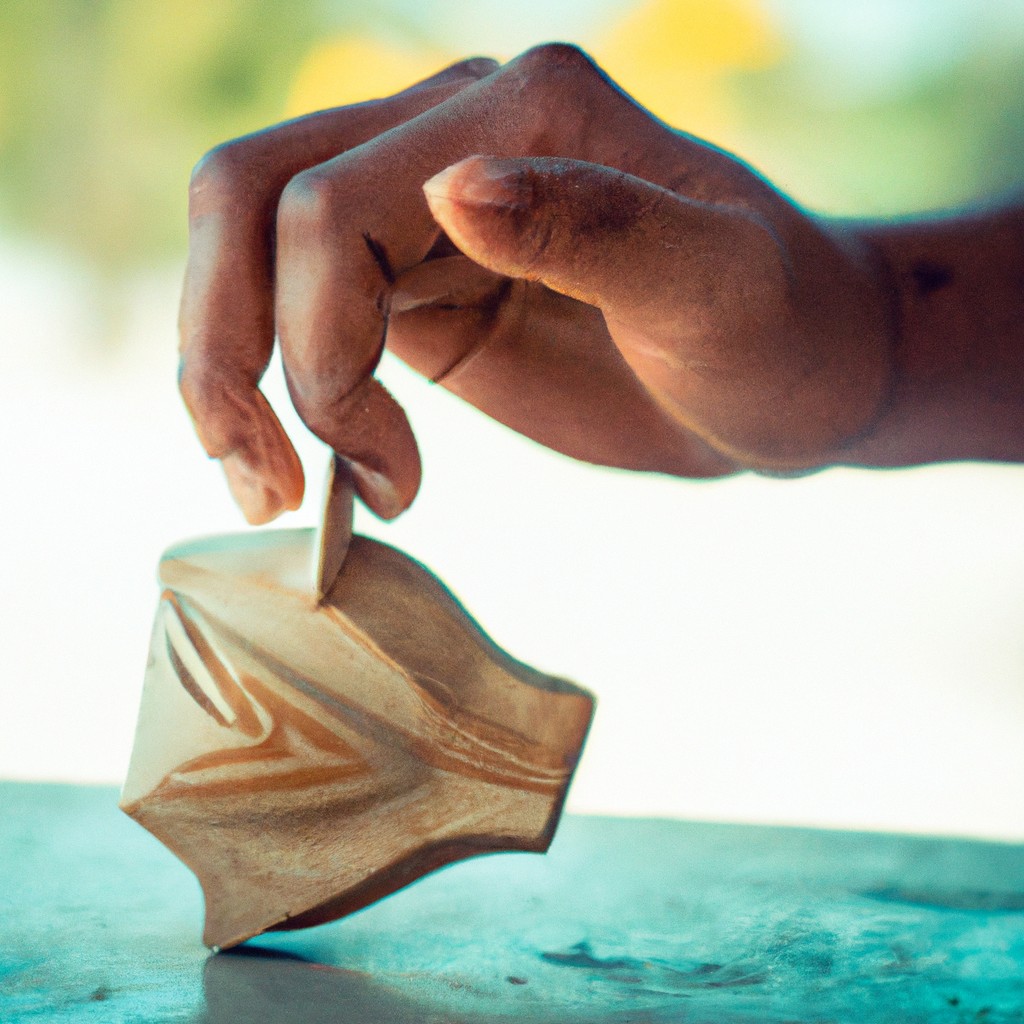
Skill development through education involves practical learning experiences, such as hands-on activities and simulations. These methods enhance understanding by engaging students actively. Teachers use various techniques to promote skill acquisition, like peer collaboration and real-world projects. By applying these strategies, students can develop essential skills that are relevant and applicable in today's fast-paced world. Furthermore, educators provide constructive feedback to guide students towards improvement and mastery. Skill development through education aims to empower individuals with the competencies needed to succeed in their personal and professional lives. Through a combination of methods and techniques, students can build expertise and confidence in their abilities.
Read more
Importance of government interventions
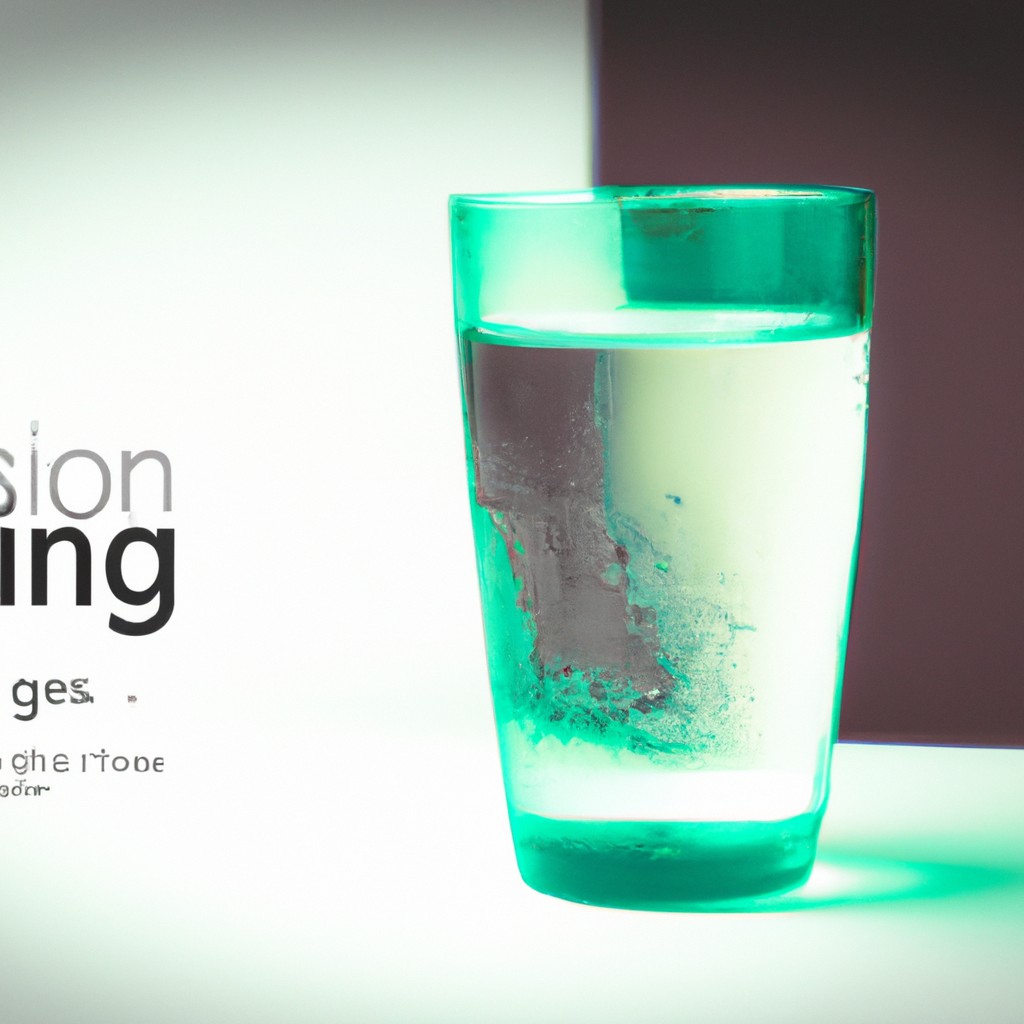
Government interventions play a vital role in safeguarding public welfare and fostering economic stability. By implementing regulations and policies, authorities can address societal inequities and promote fair competition in markets. These interventions aid in managing crises, such as natural disasters or economic downturns, providing assistance to those in need and ensuring a safety net for vulnerable populations. Government involvement can also enhance infrastructure development, healthcare accessibility, and environmental protection, creating a better quality of life for citizens. Through effective governance, interventions contribute to shaping a more equitable and prosperous society, where opportunities are accessible to all, fostering a sense of community and shared responsibility.
Read more
Importance of education in skills development
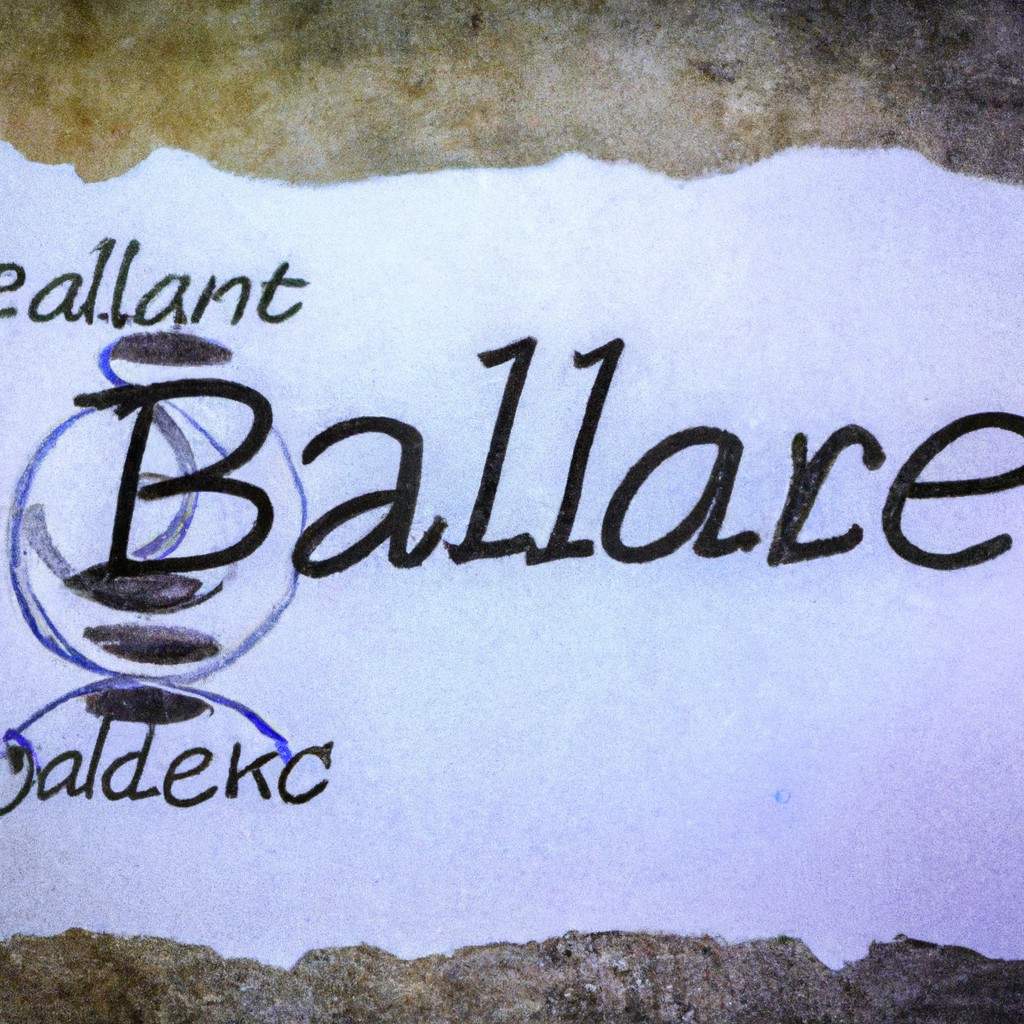
Education plays a vital role in shaping individuals' skills. Through learning, people acquire knowledge and abilities necessary for personal and professional growth. Education creates opportunities for honing critical thinking, problem-solving, and communication skills. It empowers individuals to adapt to changing circumstances and challenges in today's dynamic world. Moreover, education instills a sense of confidence and self-esteem in individuals, enabling them to pursue their goals and aspirations. By fostering continuous learning and development, education cultivates a mindset of innovation and creativity. Ultimately, education is the key to unlocking one's full potential and achieving success in various aspects of life.
Read more
Impact of government interventions on the economy
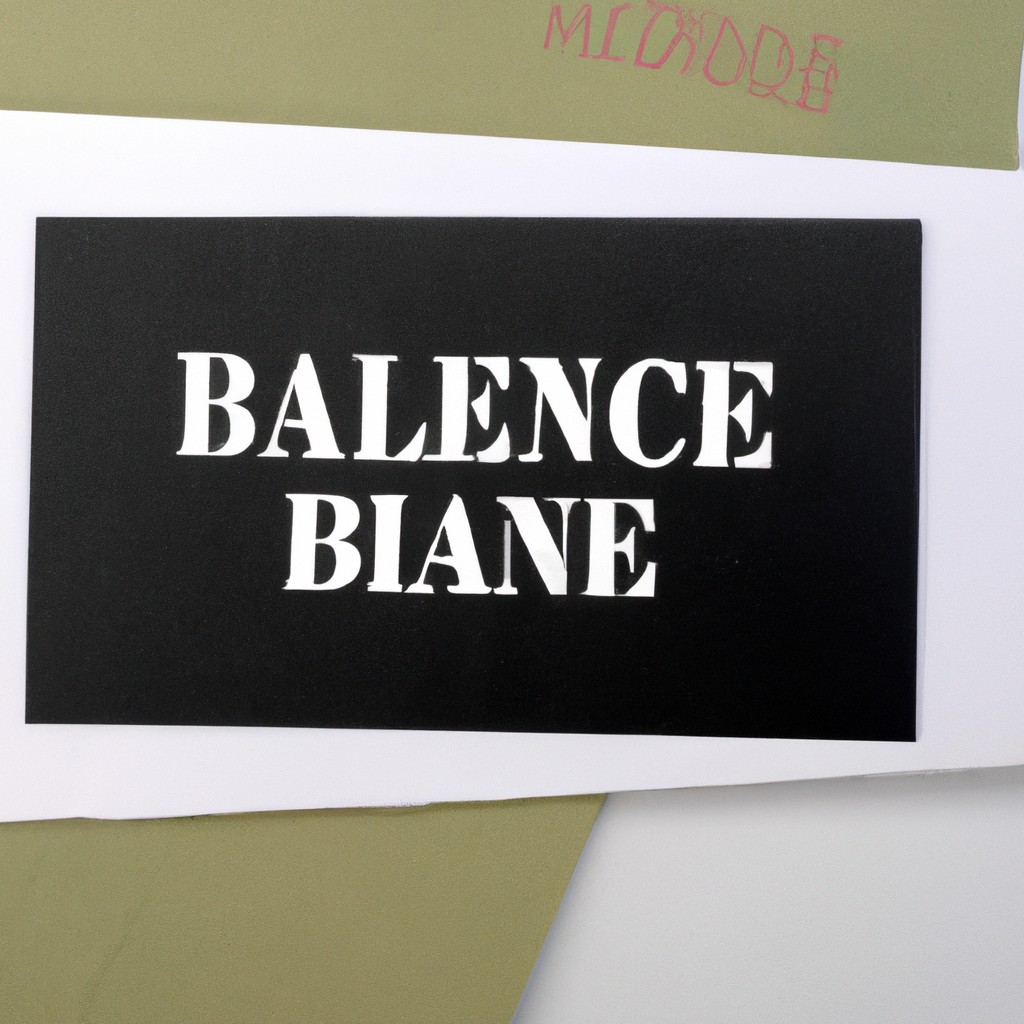
Government interventions impact the economy by shaping policies affecting businesses. These regulations influence market stability. Stimulus packages can boost economic growth during downturns. Changes in taxes and trade policies impact consumer spending. Government spending affects employment rates and inflation. Regulatory reforms can encourage or hamper business investments. Public infrastructure projects create jobs and stimulate economic activity. Subsidies can support struggling industries or distort market competition. Effective government interventions play a crucial role in maintaining a healthy economy. However, excessive interference may result in inefficiencies and market distortions. Balancing government involvement with market forces is essential for sustainable economic growth and prosperity.
Read more
Future trends and predictions for government interventions.
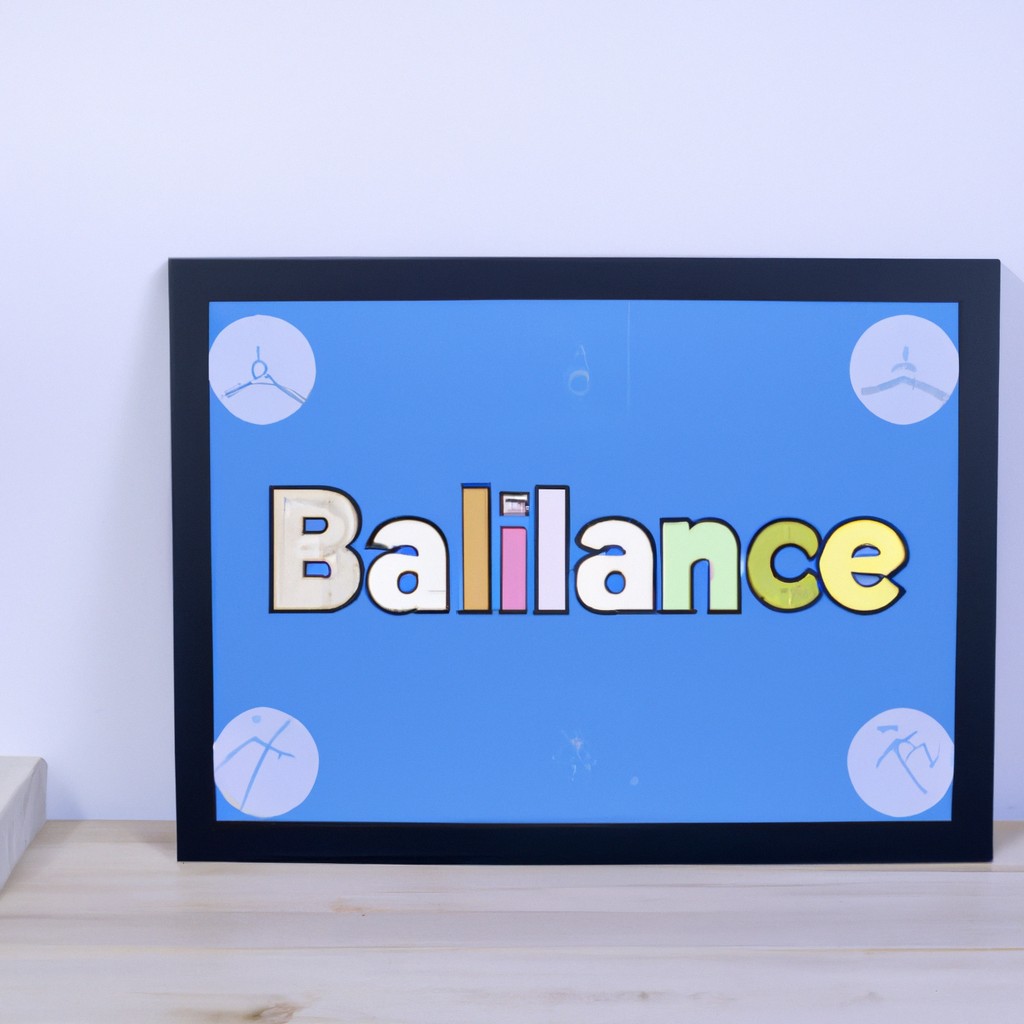
As society progresses, government interventions will increasingly focus on sustainability and technology integration. Policies may prioritize renewable energy development and smart city initiatives, enhancing quality of life. Collaborations with private sectors could drive innovation in healthcare, transportation, and education. Transparent governance and accessible digital services are likely to improve citizen engagement. Emphasis on social equity could lead to more inclusive programs and fairer distribution of resources. Continuous evaluation and adaptation of interventions will ensure relevance and effectiveness. The evolution of government interventions will shape a future where communities thrive through proactive and adaptive governance strategies.
Read more
Effective time management for skill development
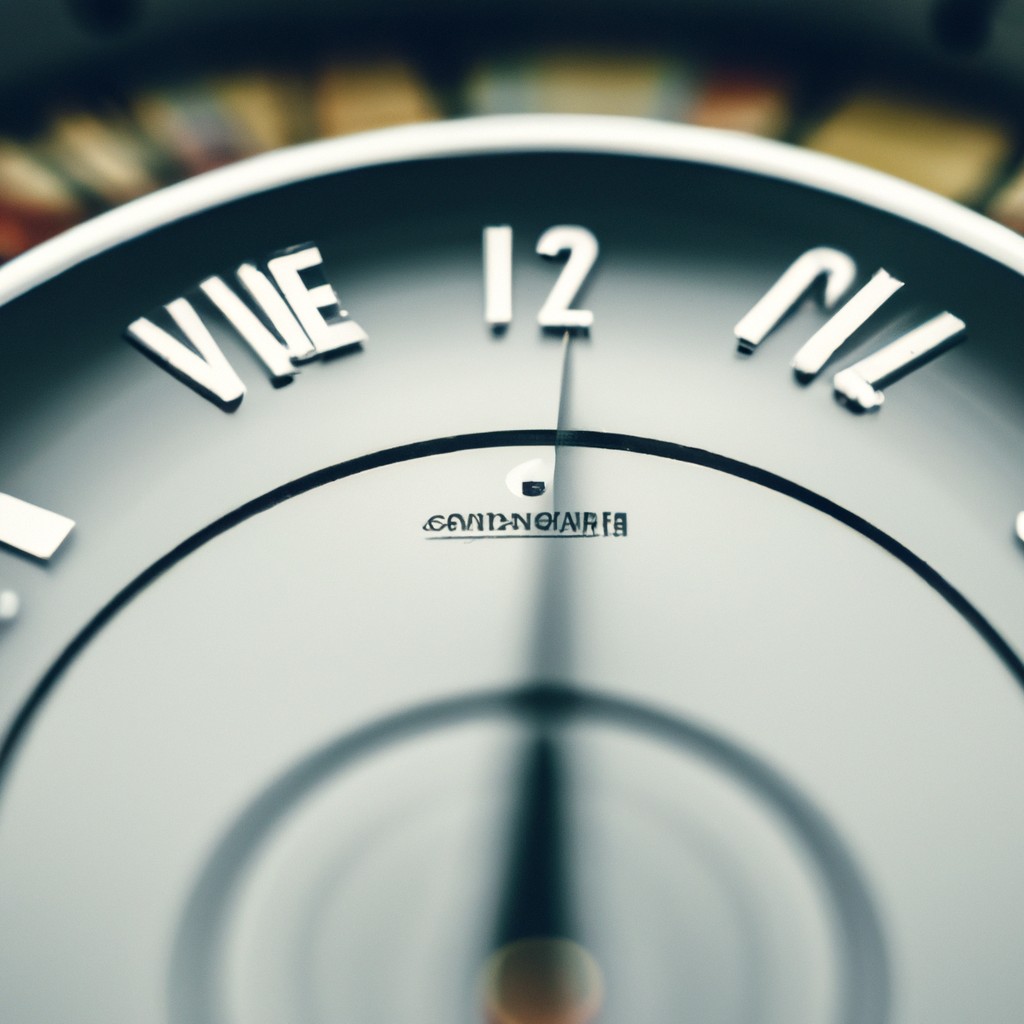
Achieving skill development requires scheduling practice into daily routines. Allocate focused blocks of time for learning. Set specific goals to measure progress and stay motivated. Prioritize tasks based on importance. Use tools like planners to organize activities efficiently. Avoid multitasking to enhance concentration. Break tasks into smaller achievable steps. Reflect on accomplishments regularly to track improvement. Seek support and guidance from mentors. Stay consistent and disciplined in time management efforts. Embrace the process of growth and skills enhancement. Remember that consistent effort leads to significant skill development success. Enjoy the journey of self-improvement through effective time management strategies.
Read more
Different learning methods
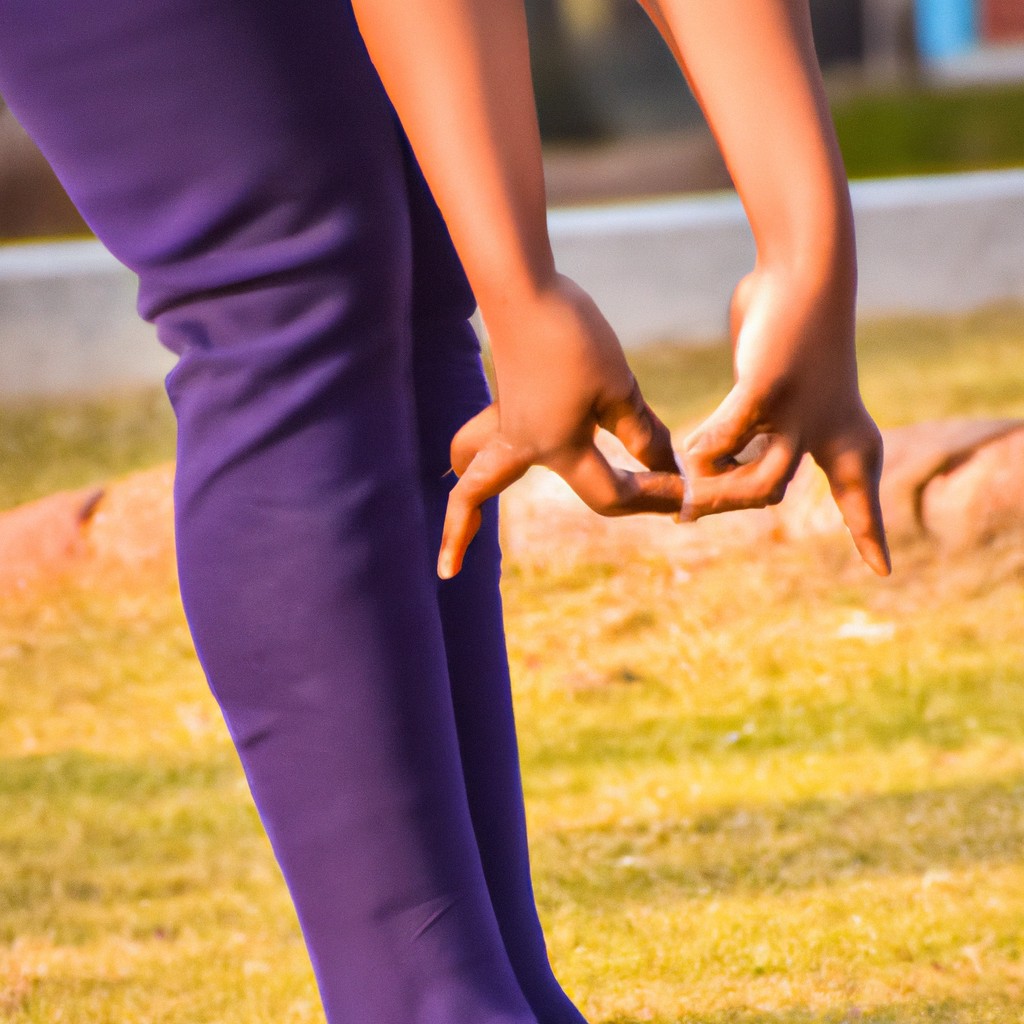
Students learn through diverse methods like visual aids, hands-on experiences, discussions, and multimedia resources. These multiple approaches cater to various learning styles, ensuring effective comprehension and retention. Visual learners prefer diagrams and charts to grasp information quickly, whereas kinesthetic learners benefit from practical applications to reinforce concepts. Auditory learners thrive in discussions and lectures, absorbing information through listening. Utilizing a combination of these methods can enhance the learning experience, making it more engaging and accessible to all learners. Understanding and adapting to different learning styles can promote inclusivity, creativity, and successful learning outcomes for individuals in various educational settings.
Read more
Challenges and criticism of government interventions
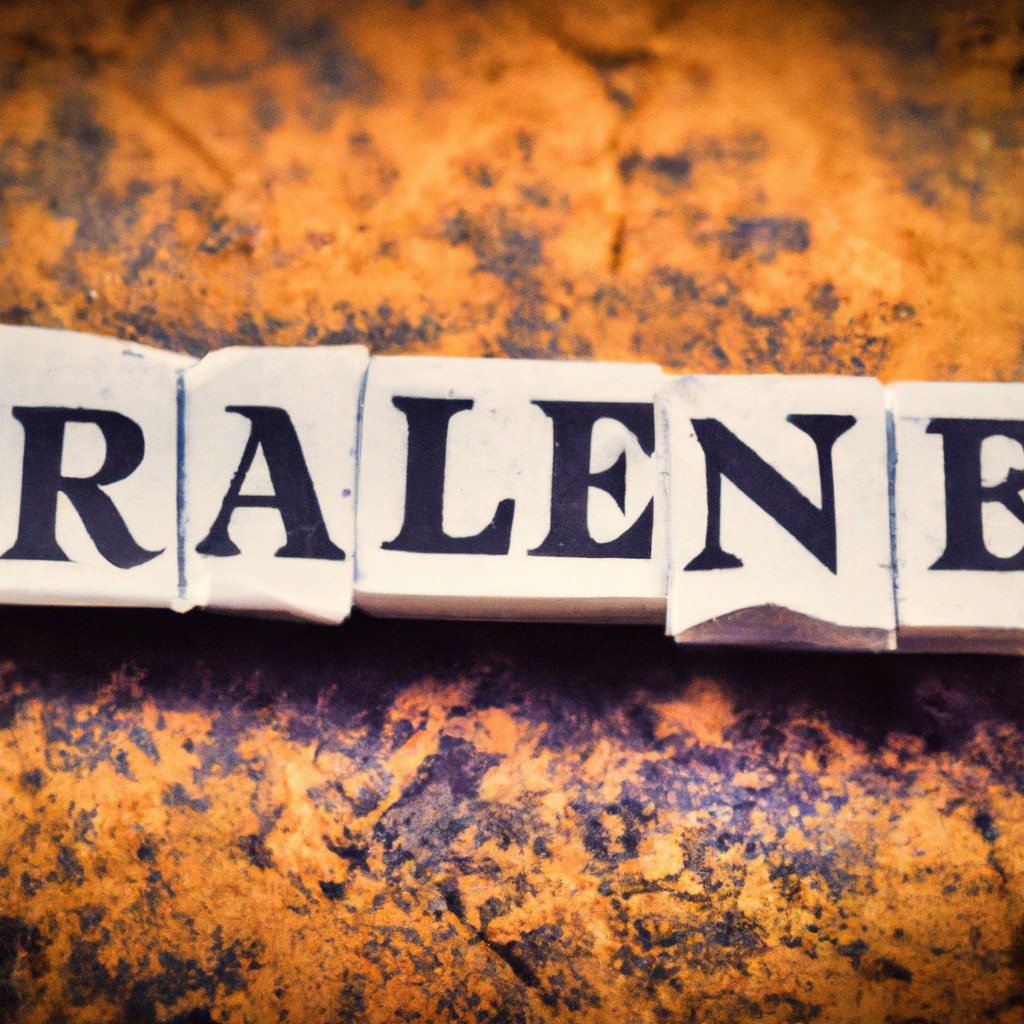
Government interventions face scrutiny due to lack of transparency, potential inefficiencies, and unintended consequences. Criticism often centers on the effectiveness of policies and their impact on individual freedoms. Challenges arise when interventions lack public support or fail to address underlying issues effectively. Critics argue that government overreach can stifle innovation and economic growth, leading to dependency. Balancing the need for intervention with respect for personal liberties is a delicate task for policymakers. Public discourse plays a crucial role in shaping government actions, holding officials accountable and promoting democratic values. Addressing these challenges requires open dialogue and a commitment to continuous improvement.
Read more
Benefits of skill development through education.
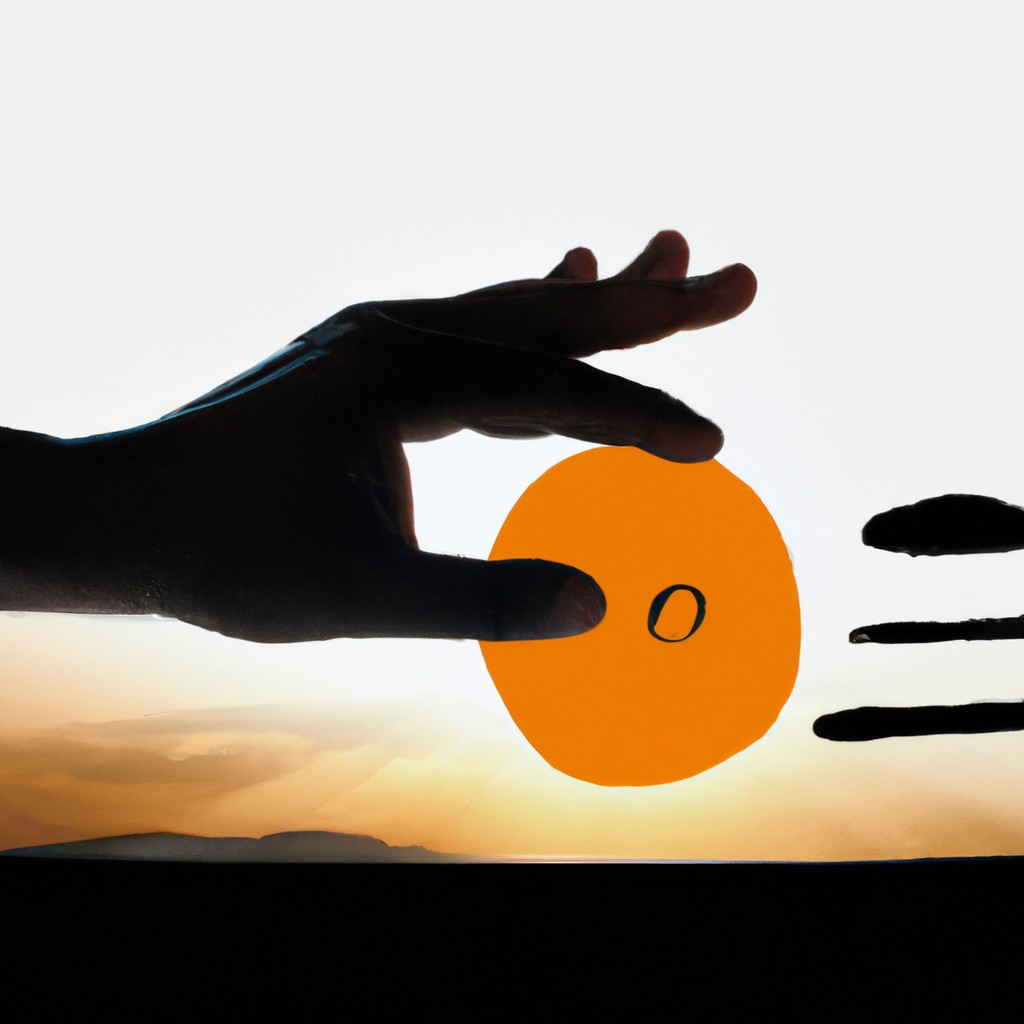
Skill development through education offers individuals the opportunity to enhance their expertise and capabilities significantly. This process enables them to acquire essential knowledge and practical skills that are crucial for their personal and professional growth. By fostering a continuous learning mindset, education empowers individuals to adapt to new challenges and seize rewarding opportunities in various fields. Through structured learning experiences, individuals can cultivate leadership traits, critical thinking abilities, and effective communication skills, all of which are invaluable assets in today's competitive world. Education also nurtures creativity and innovation, encouraging individuals to think outside the box and pursue their ambitions with confidence.
Read more
Benefits of acquiring new skills
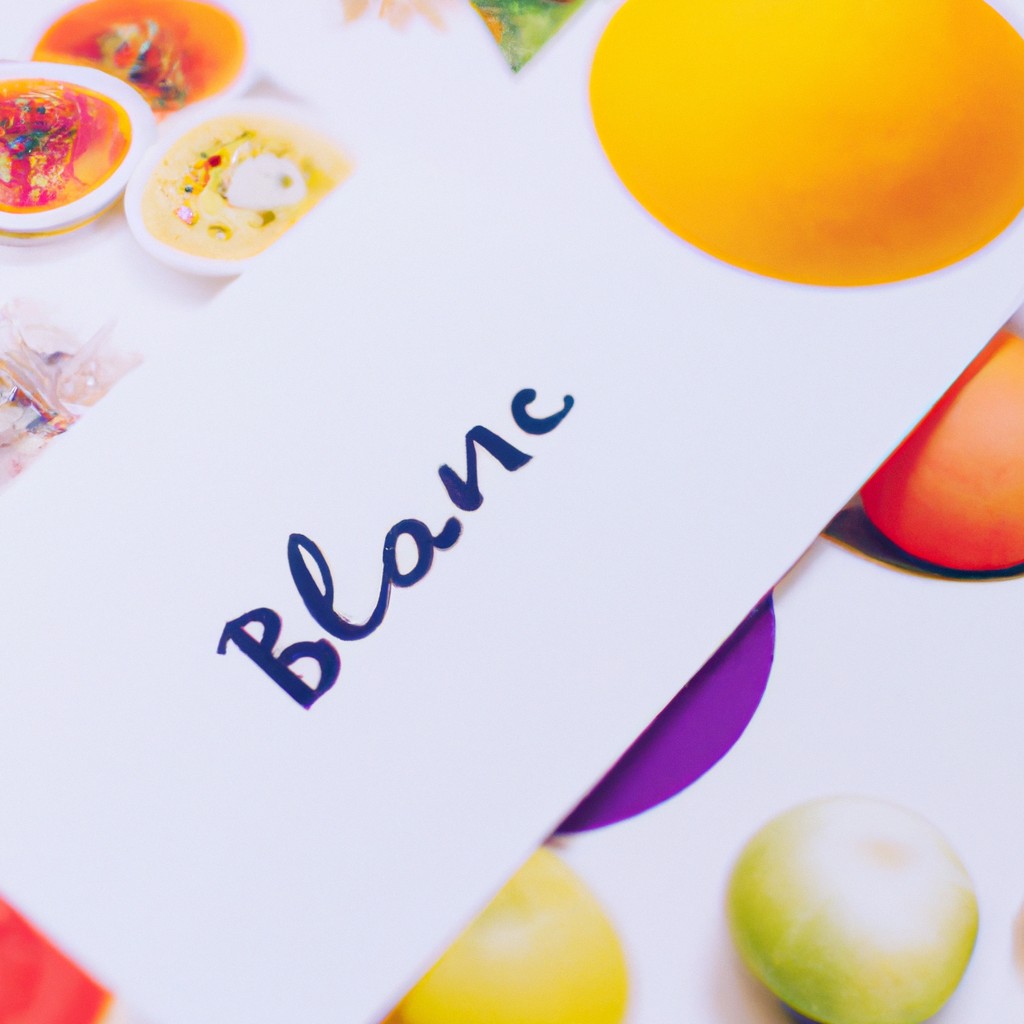
Acquiring new skills enhances personal growth, boosts confidence, opens new opportunities, and improves employability. Developing diverse abilities equips individuals with adaptability and resilience in an ever-changing world. Learning stimulates creativity, ignites curiosity, and fosters a sense of accomplishment and satisfaction. Mastering new skills enables individuals to enrich their lives, expand their perspectives, and discover hidden talents. Continuous learning promotes mental sharpness, increases self-esteem, and provides a sense of purpose. Embracing new challenges cultivates a growth mindset and enhances problem-solving skills. By acquiring new skills, individuals not only invest in themselves but also contribute positively to their communities and society as a whole.
Read more