Non-Response and Selection Bias
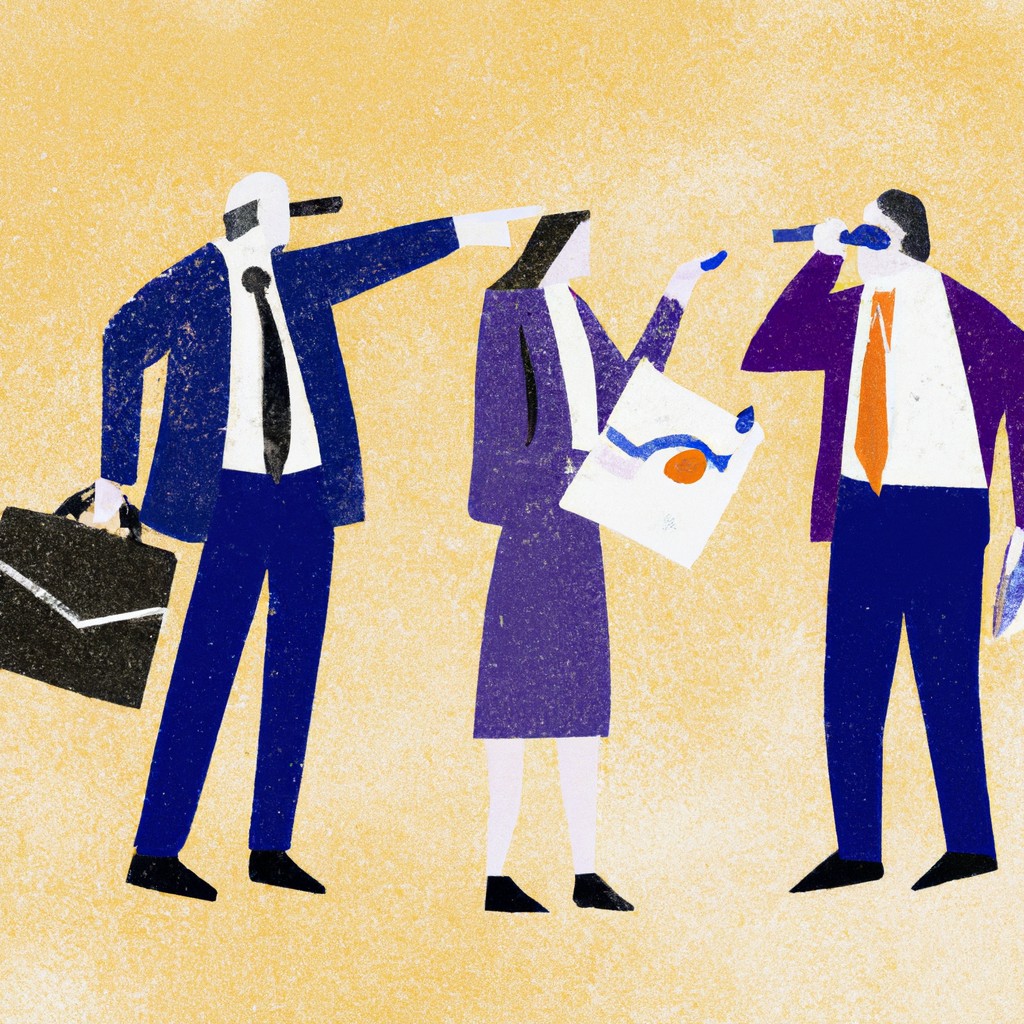
Non-response and selection bias can significantly impact the validity of research findings. When individuals choose not to participate in a study, the sample may not accurately represent the population. This can lead to skewed results and erroneous conclusions. Researchers must consider the reasons for non-response and implement strategies to minimize selection bias. By understanding the implications of non-response, researchers can adjust their methodologies to address potential biases. Effective communication and outreach efforts can improve response rates and reduce the impact of selection bias on study outcomes. Overall, addressing non-response and selection bias is crucial for ensuring the reliability and relevance of research findings.
Read more
Limitations of the Gini Coefficient
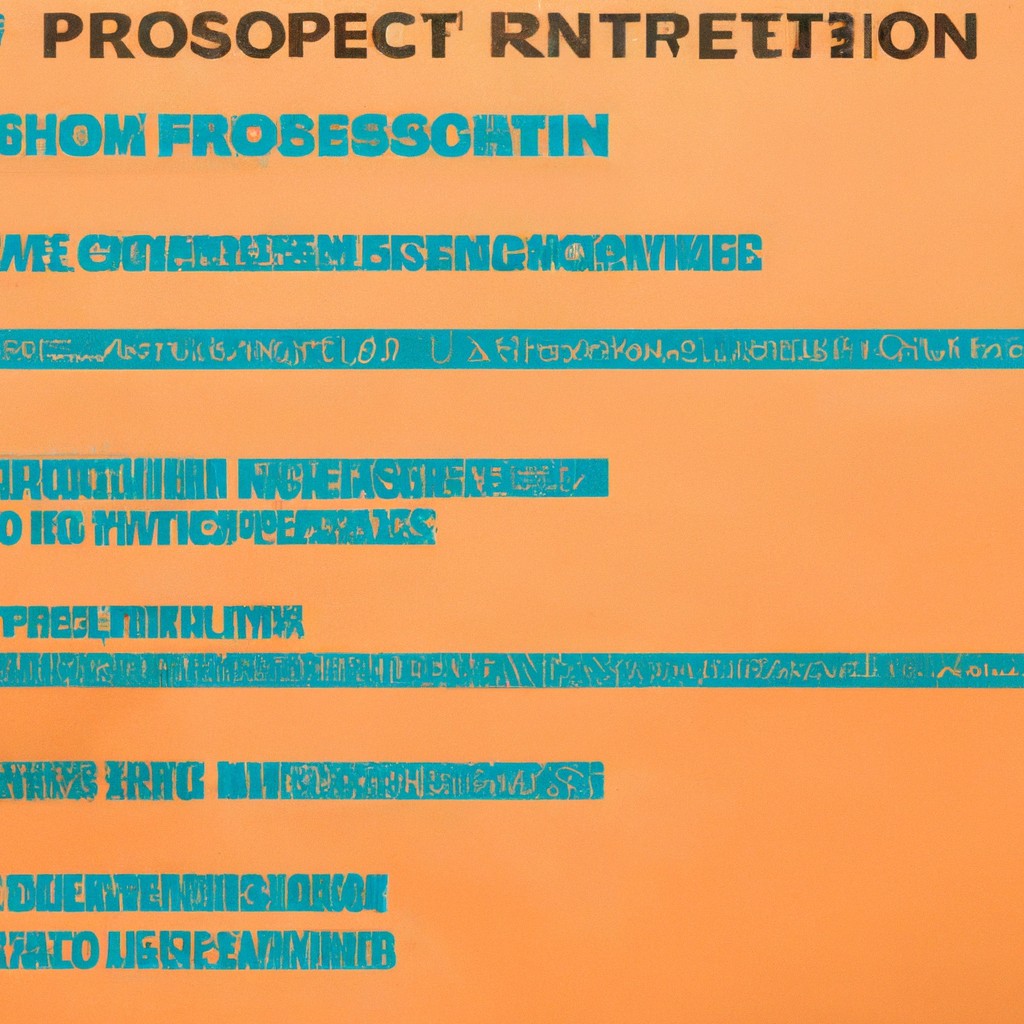
The Gini coefficient is criticized because it fails to account for income distribution nuances. It might oversimplify complex economic disparities and overlooks the impacts of discrimination and societal structures. This metric does not distinguish between various sources of income, like wages, capital gains, or inheritance. It also disregards non-monetary factors influencing individual well-being, such as access to healthcare or education. Critics argue that the Gini coefficient masks inequalities within specific demographic groups or regions. Policymakers should use caution when relying solely on this measure to assess inequality and design interventions. Further research and supplementary metrics can provide a more comprehensive understanding of economic disparities.
Read more
Calculation method of the Gini Coefficient
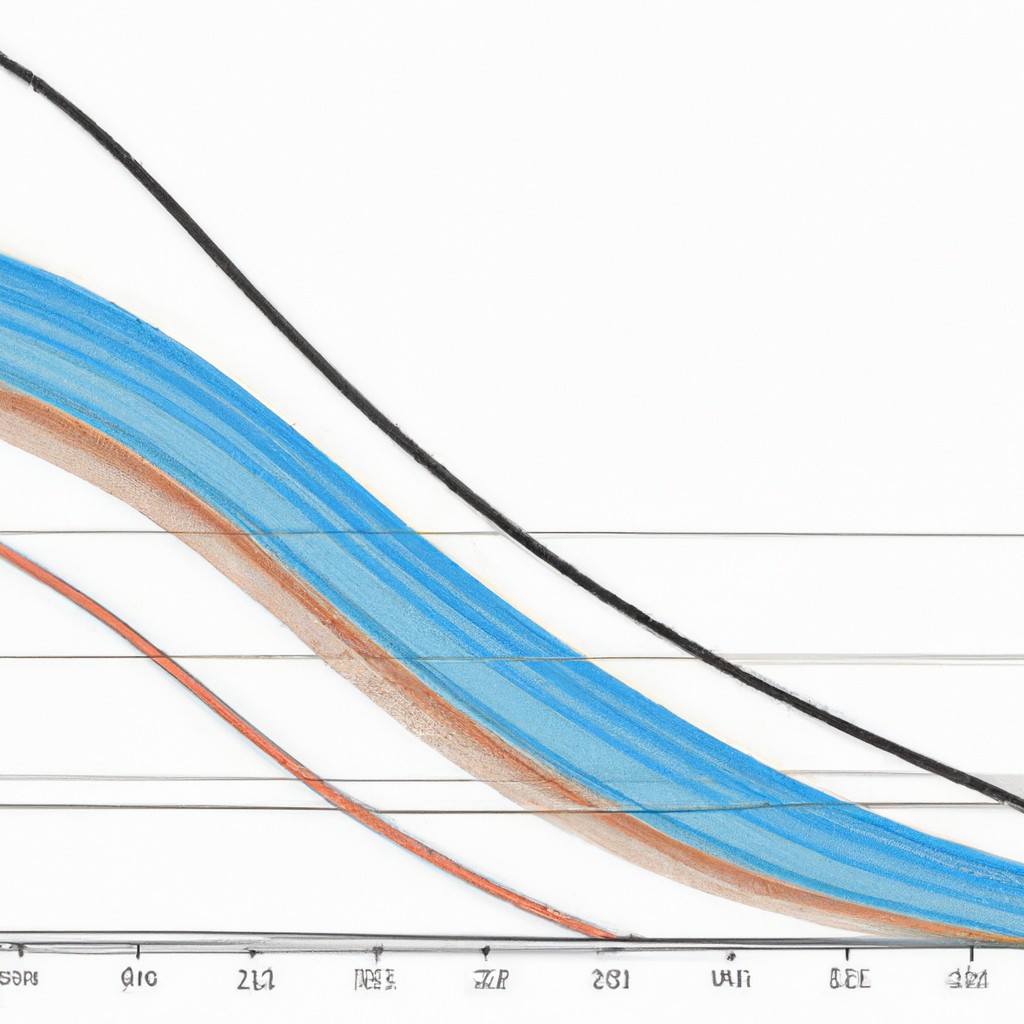
The Gini coefficient calculates income inequality. It ranges from 0 to 1, where higher values indicate more inequality. To compute the Gini coefficient, first, arrange individuals by income. Next, create a Lorenz curve plotting cumulative income against cumulative population. The Gini coefficient is the area between the Lorenz curve and the line of perfect equality, divided by the total area under the line of perfect equality. A coefficient of 0 indicates perfect equality, while 1 shows maximum inequality. Governments and policymakers utilize the Gini coefficient to assess and address wealth disparities within a society, aiming for more equitable distribution of resources.
Read more
Bias and Sampling Error
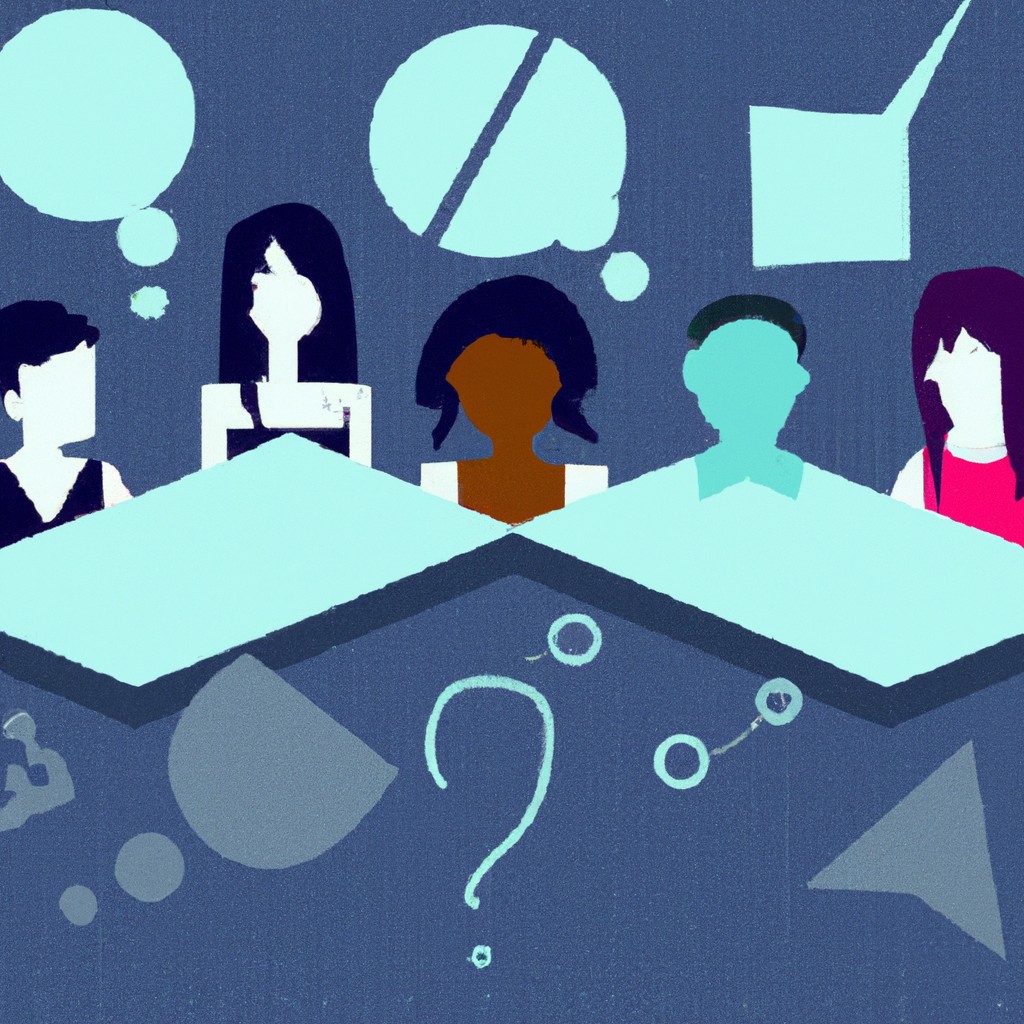
Bias and sampling error can affect the accuracy of research findings. Bias occurs when the data collection process is flawed, leading to incorrect results. Sampling error, on the other hand, arises from using a sample that does not accurately represent the population. Both can lead to misleading conclusions and impact decision-making. It's crucial to understand these concepts to evaluate the reliability of research studies. Researchers must strive to minimize bias and sampling error to ensure the validity of their findings. By being aware of these factors and implementing proper methodologies, researchers can enhance the quality and credibility of their research outcomes.
Read more
Application of the Gini Coefficient in different countries
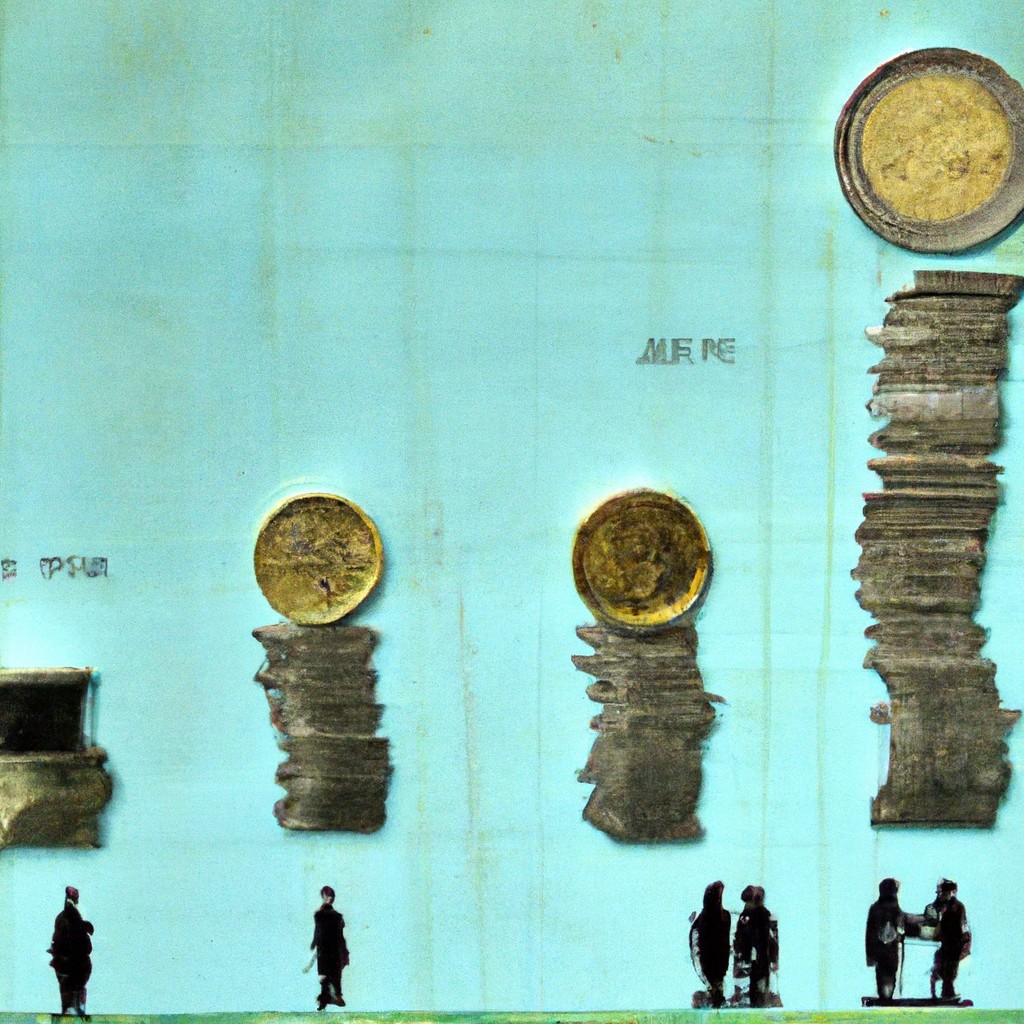
The Gini Coefficient gauges income inequality. Lower figures reflect more equality, with zero depicting total equality. Various nations utilize this metric to examine societal disparities and craft policies fostering equitable wealth distribution. In Sweden, a low coefficient indicates a more equal society, supported by progressive taxation and robust social welfare programs. South Africa faces high inequality, influenced by a history of apartheid and economic disparities. China's coefficient has risen due to rapid economic growth, yet rural-urban divisions persist. Each country's unique context shapes the Gini Coefficient's application and highlights the ongoing global challenge of addressing income inequality.
Read more
Measurement approaches
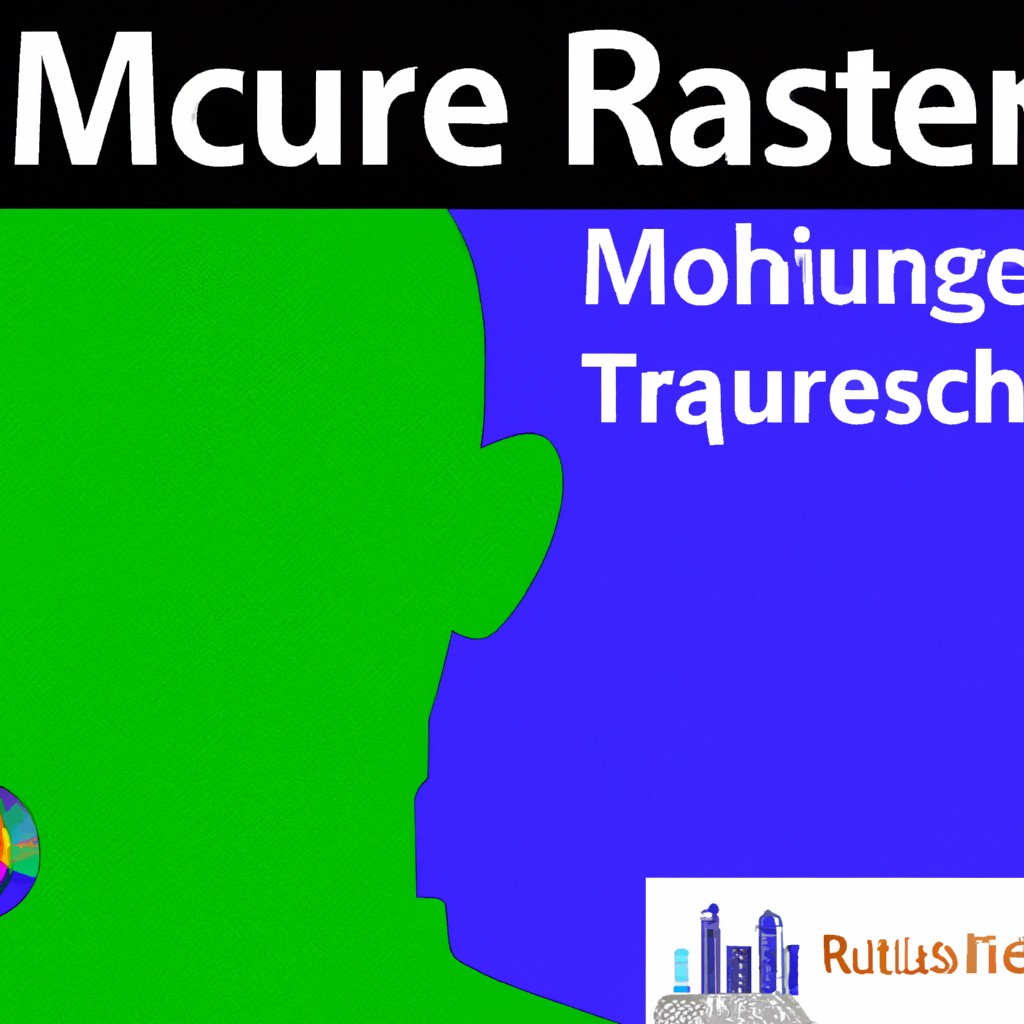
Measurement approaches play a crucial role in gathering accurate data for analysis. Utilizing multiple methods enhances data reliability. Measuring variables through quantitative surveys aids in statistical analysis. Qualitative interviews deepen understanding through detailed insights. Observational approaches provide real-time data on behaviors. Combining different measurement techniques can offer a comprehensive view of the phenomenon. Flexibility in approach selection ensures suitability for diverse research contexts. Effective measurements contribute to robust research outcomes and informed decision-making. Striking a balance between quantitative and qualitative methods enriches data interpretation. Continuous evaluation of measurement strategies enhances research validity and credibility. Researchers must carefully select the most appropriate measurement approaches for their study objectives.
Read more
Limitations and criticisms of Gini Coefficient
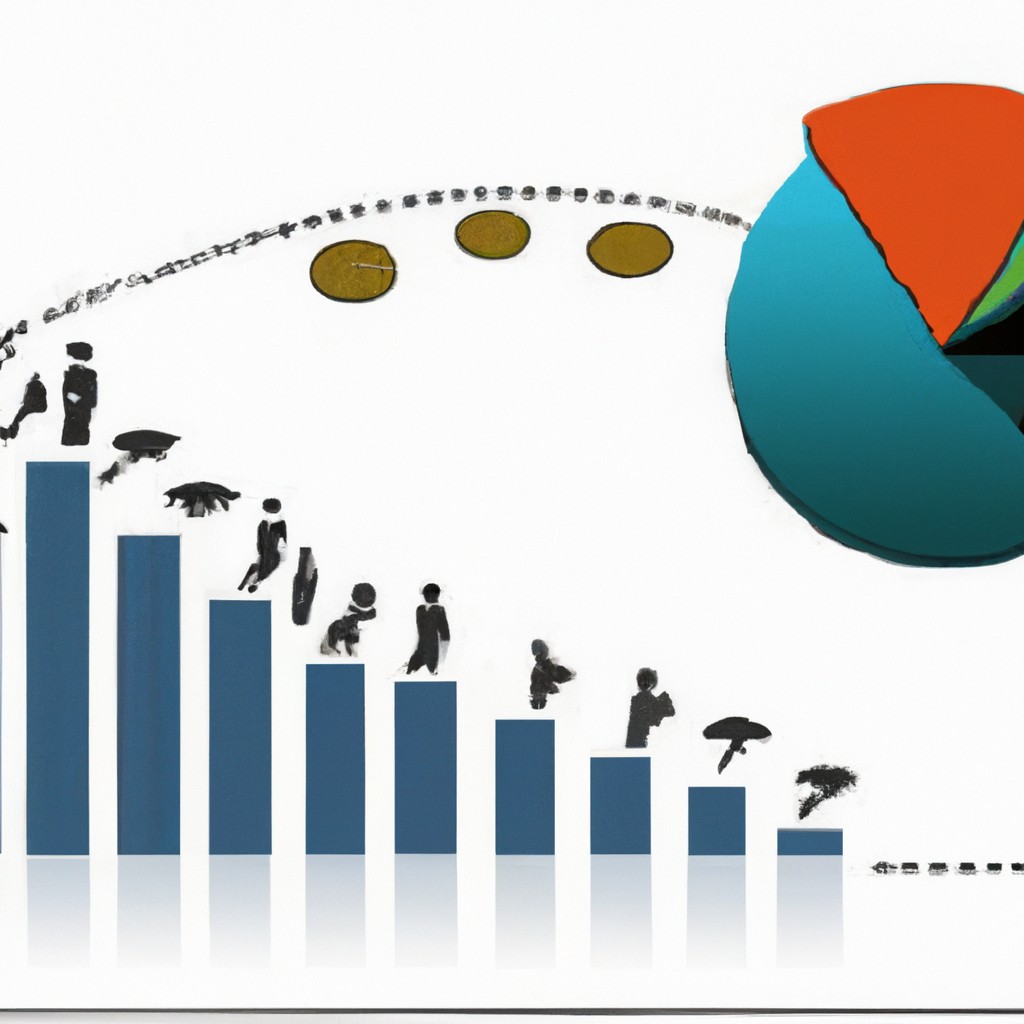
Critics argue Gini Coefficient oversimplifies income inequality.
It can't capture nuances of wealth distribution.
The measure doesn't address factors like social mobility.
Divides society into income brackets, overlooking individual circumstances.
Critics say Gini overlooks wealth concentration at the top.
It underestimates growing disparities in high-income groups.
The metric may not reflect true economic disparities accurately.
Some argue it lacks sensitivity to redistribution policies' impacts.
Critics find it insufficient for capturing inequalities within groups.
There are concerns about its use in comparing different populations.
In conclusion, experts call for a comprehensive approach beyond Gini for income inequality analysis.
Read more
Importance and applications of Gini Coefficient
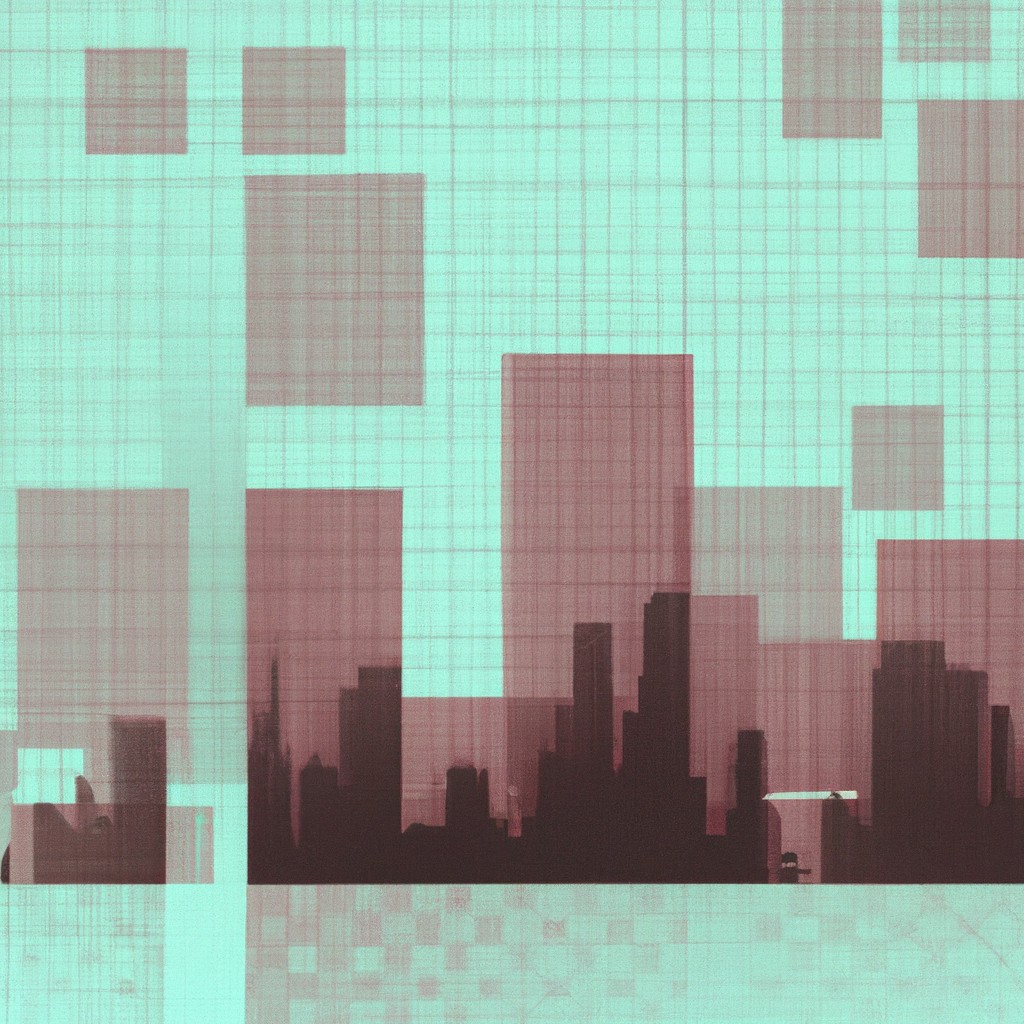
The Gini Coefficient measures income inequality, aiding policymakers in designing effective social welfare programs. It evaluates fairness in income distribution, guiding efforts to reduce disparities among individuals. Applied in economics, sociology, and policy analysis, the Gini Coefficient highlights disparities and informs strategies for equitable wealth distribution. By capturing social and economic inequalities, it enhances societal understanding of wealth gaps and promotes inclusive development. Policymakers rely on the Gini Coefficient to monitor progress in combating poverty and fostering social justice. Its versatility in various disciplines underscores its significance in shaping inclusive policies and fostering economic prosperity for all.
Read more
History of the Gini Coefficient
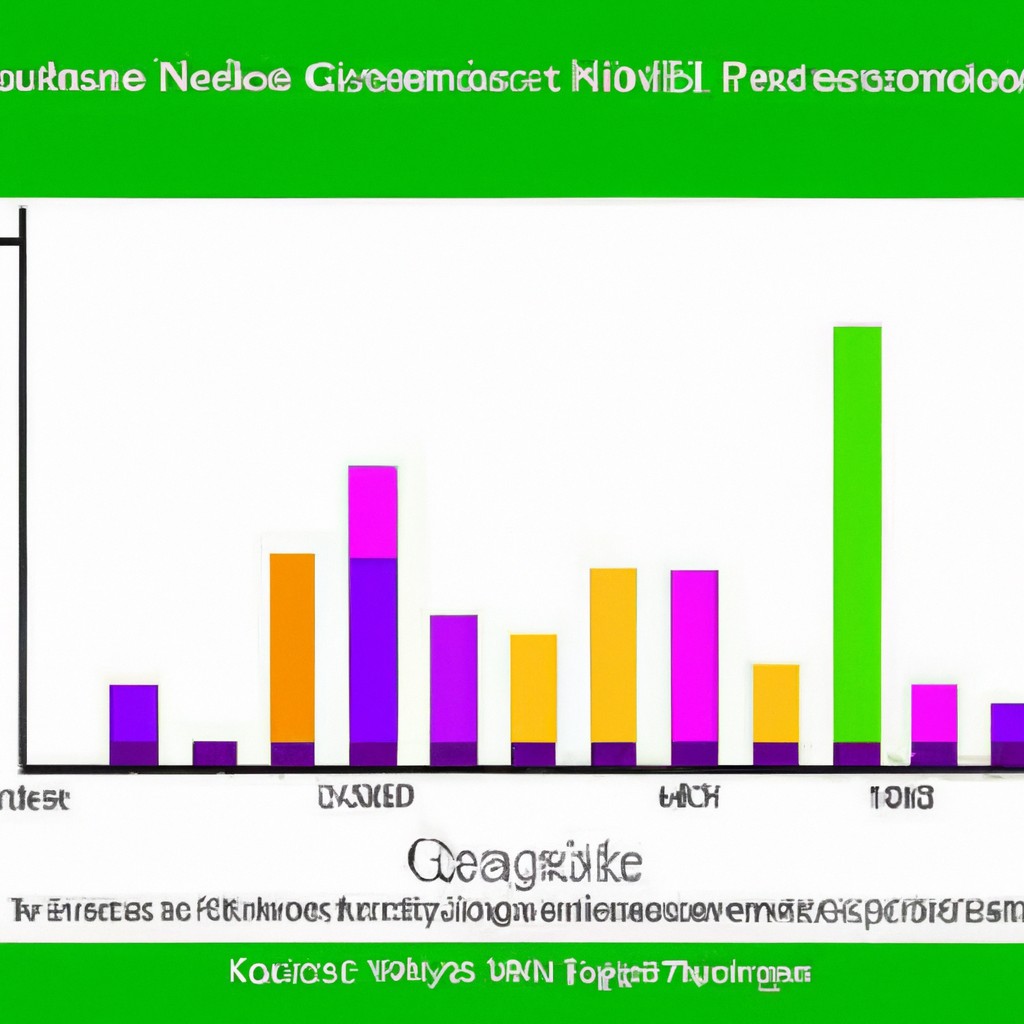
The story of the Gini Coefficient began in 1912 when Italian statistician Corrado Gini first introduced it. Gini developed this measure to track income distribution within a population, aiming to capture inequality levels effectively. Over time, it gained popularity globally, becoming a vital tool in analyzing societal disparities. Economists widely adopted the Gini Coefficient due to its simplicity and robustness. The coefficient ranges from 0 (perfect equality) to 1 (maximum inequality), offering insight into wealth gaps. Despite criticisms, it remains a key indicator in economic research, shaping policy decisions and social interventions worldwide. The Gini Coefficient continues to evolve, aiding in understanding and addressing income inequality.
Read more
Historical background of Gini Coefficient
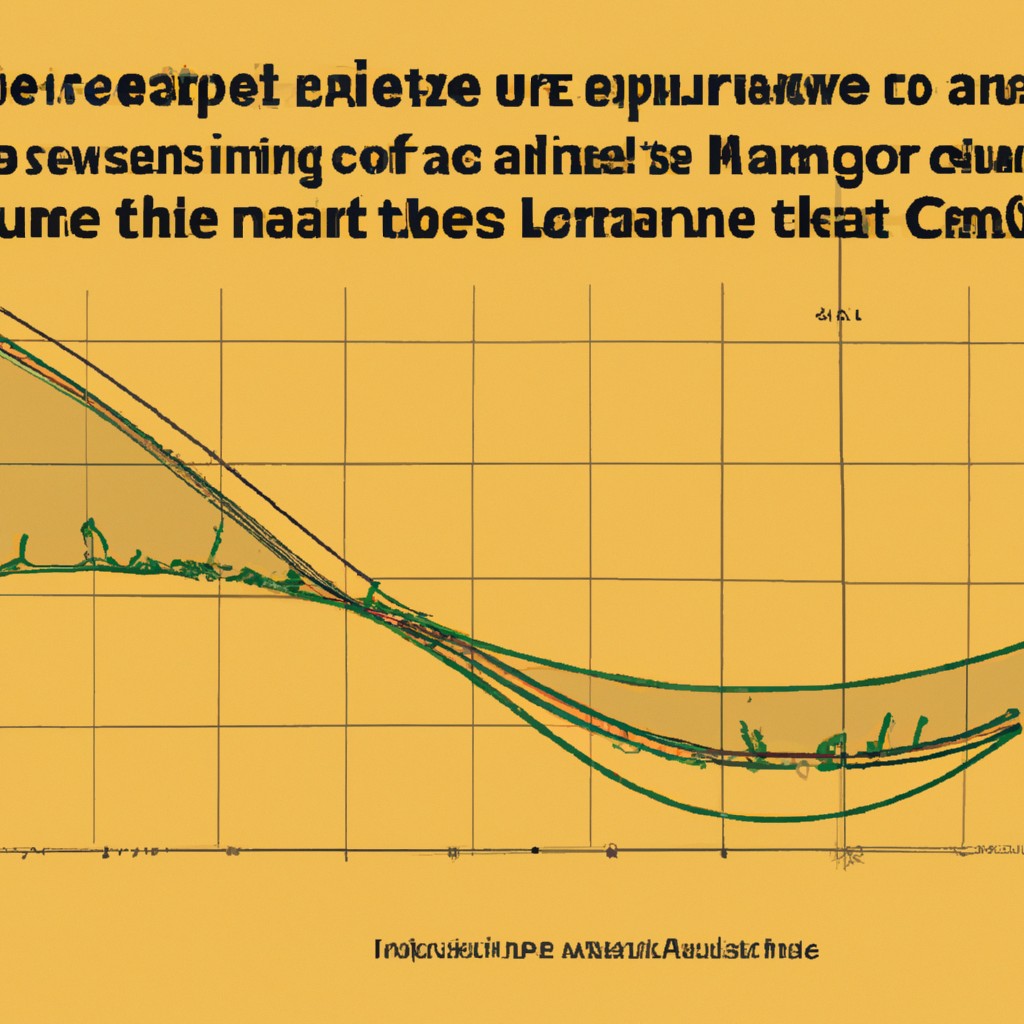
The Gini coefficient, developed by Italian statistician Corrado Gini in 1912, measures income inequality distribution. It ranges from 0 (total equality) to 1 (total inequality). Gini's formula became widely used after World War II to assess economic disparities. By highlighting disparities, governments could implement policies to address social inequality. Its simplicity and effectiveness enabled global comparisons, enhancing academic research and policymaking. Widely referenced in historical and sociopolitical literature, the Gini coefficient remains a vital tool for understanding economic and social dynamics. Its enduring relevance reflects ongoing global efforts to promote equity and justice for all individuals.
Read more